29 March 2023
Data Driving Clever Transport
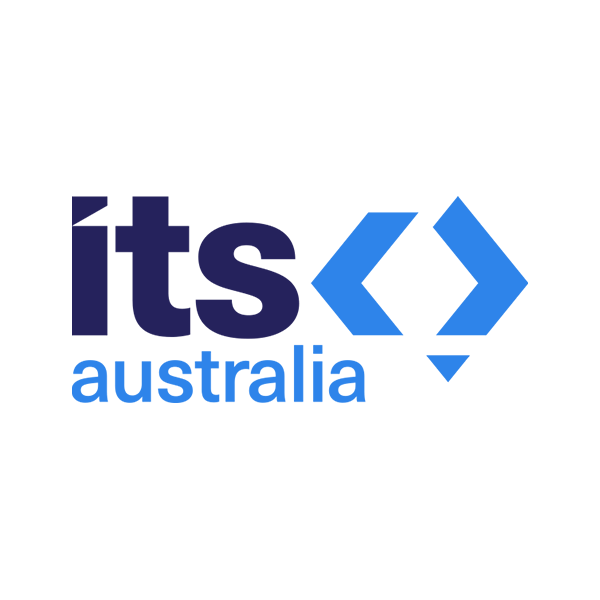
ITS Australia
The panel discussion dives into how data can be used to create more efficient, sustainable, and intelligent transportation systems, including the use of real-time data, predictive analytics, and machine learning.
Panellists
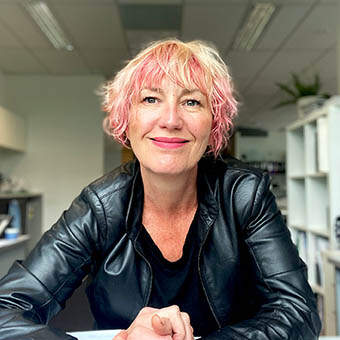
Stacey Ryan (Moderator)
Policy Manager / ITS Australia
Stacey brings to ITS Australia more than 20 years’ experience in communications, policy, and engagement in the public and private sector. In government, she worked in highly regulated environments including transport, planning, liquor and gaming, and emergency services. Stacey has a strong policy development and legislative background and managed engagement and building strong relationships with external stakeholders across all levels of government, NGOs, clients, collaborators, and the media; fostering partnerships that collaboratively work to solve problems we collectively face.
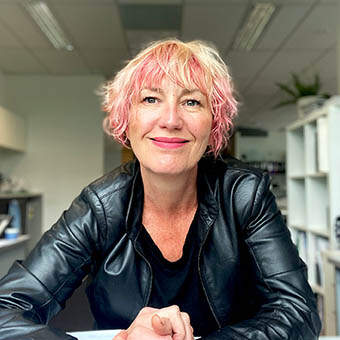
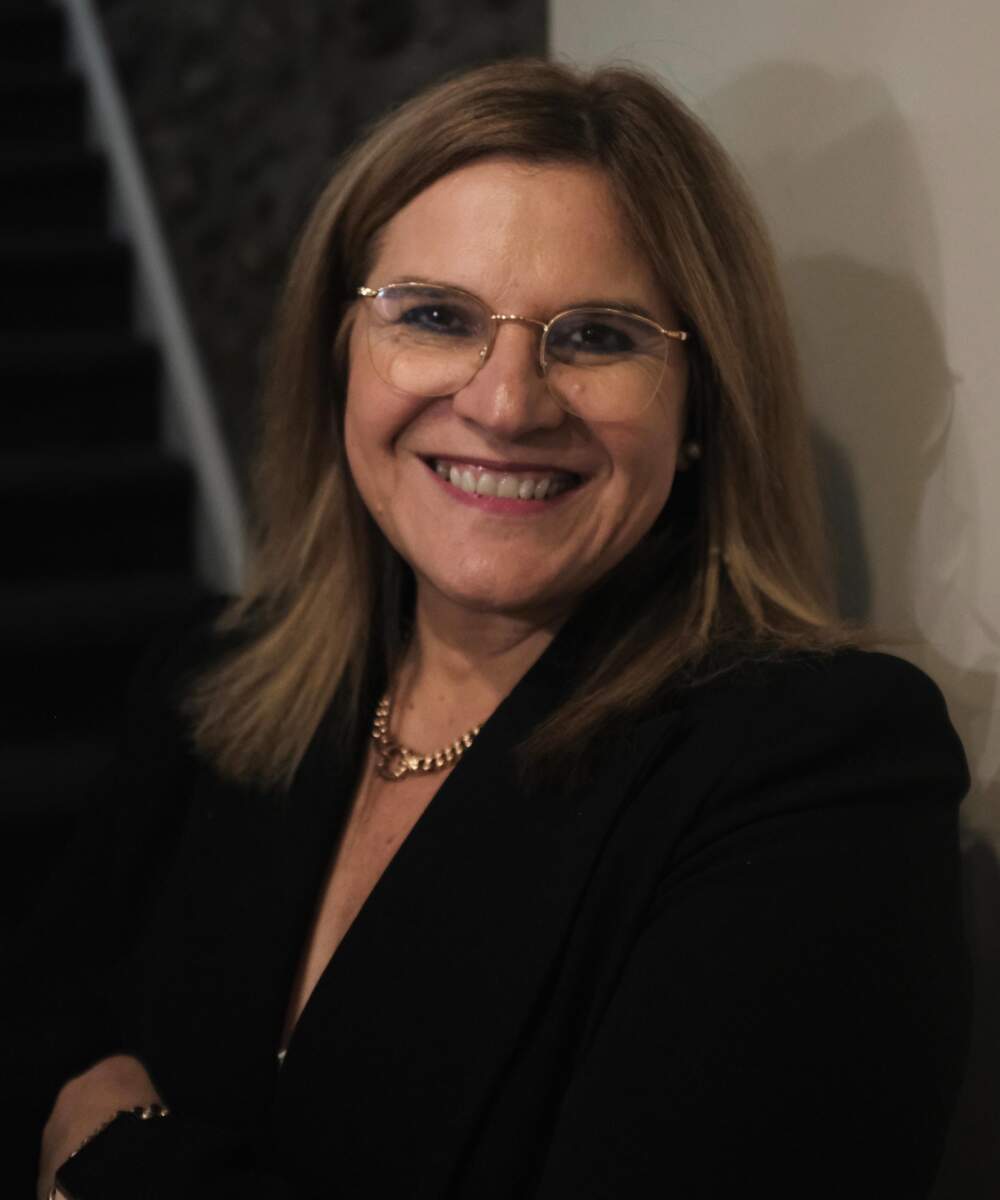
Rita Excell
Founder / Rita Excell Advisory
Rita is a qualified Civil Engineer with over 25 years’ experience across transport planning, urban planning, business transformation and managing organisational change. She is a Fellow of the Institute of Public Works Engineering Australasia and is the immediate past president of that association. Rita has been invited to be an expert contributor to Connected and Automated Vehicle steering groups across Australia, New Zealand, United States, Singapore and Canada.
Rita's extensive career has covered, local government, consulting and industry associations, including running the Australia and New Zealand Driverless Vehicle Initiative (ADV), from 2016 to 2021. She was previously the industry head for Transport across ANZO for AWS where she worked with Public Sector Customers, across Land, Sea, Air and Rail Transport.
As the key spokesperson for a number of organisations across her career, Rita has delivered keynote addresses on the topic of driverless vehicles across the globe and has met with all levels of governments and their agencies in Australia, New Zealand, United States and UK. Career highlights include establishing the International ADVI program, running the first on-road demonstration of automated vehicle technology in the Southern Hemisphere in 2015, being the first female President of the Institute of Public Works Engineering, SA and Australasia, as well as having the IPWEA scholarship for Women in asset management named after her.
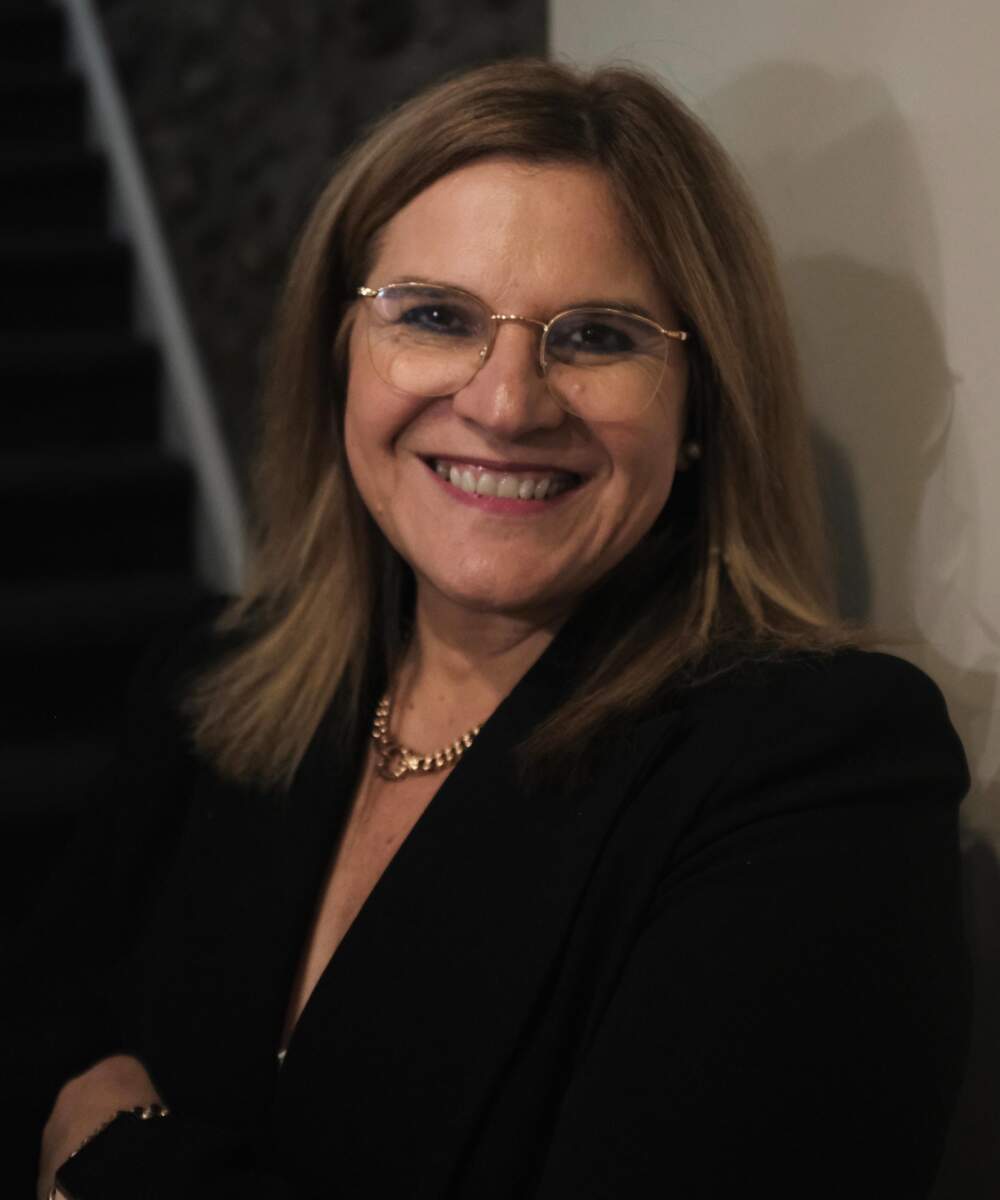
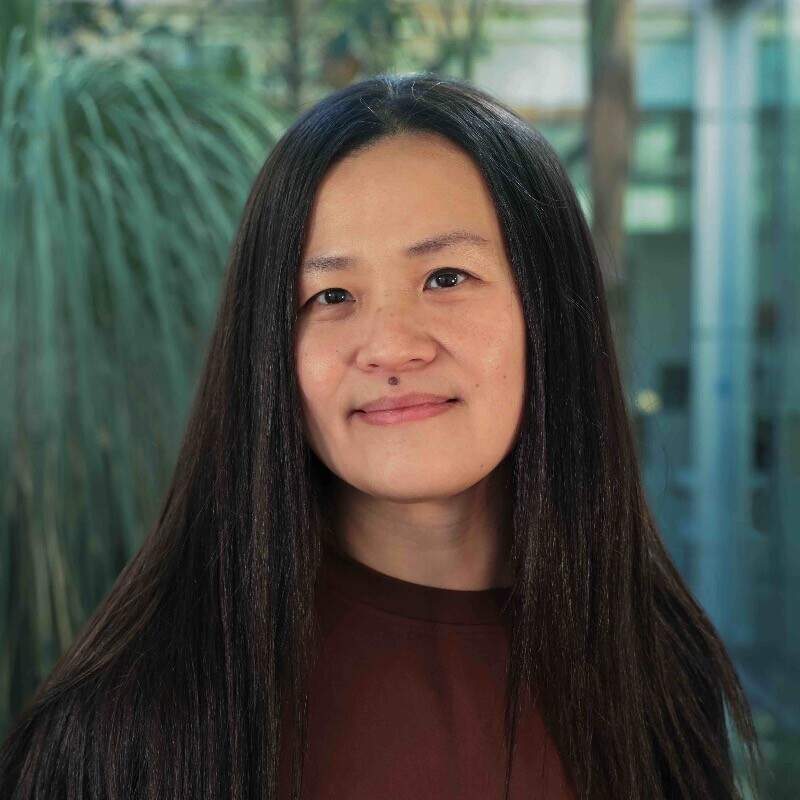
Professor Flora Salim
Professor, Deputy Director (Engagement) / UNSW AI Institute
Professor Flora Salim is a distinguished academic in the field of AI and computer science. the Deputy Director (Engagement) of the UNSW AI Institute. Her work focuses on fundamental AI methods and foundation models applicable for smart and sustainable cities, such as for mobility, transport, energy, and grid systems. She has received multiple nationally and internationally competitive fellowships, such as Humboldt Fellowship, Bayer Fellowship, and many accolades and awards such as the Women in AI Award Australia and New Zealand (2022) and IBM Smarter Planet Industry Innovation Award. Prof Salim is a Chief Investigator on the Australian Research Council (ARC) Centre of Excellence for Automated Decision Making and Society (ADM+S), co-leading the Mobilities Focus Area. She is also a Key Chief Investigator in the ARC Training Centre for Whole Life Design for Carbon Neutral Infrastructure, leading the Program on Machine Learning for Carbon Performance. She is a member of the Australian Academy of Sciences’ National Committee for Information and Computing Sciences and a member of the Australian Research Council (ARC) College of Experts.
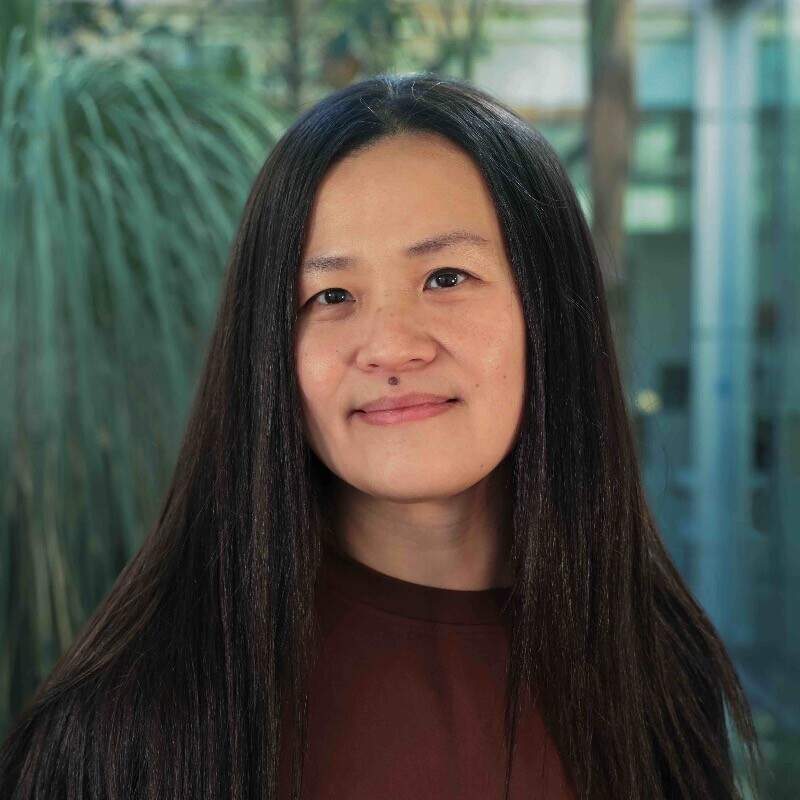
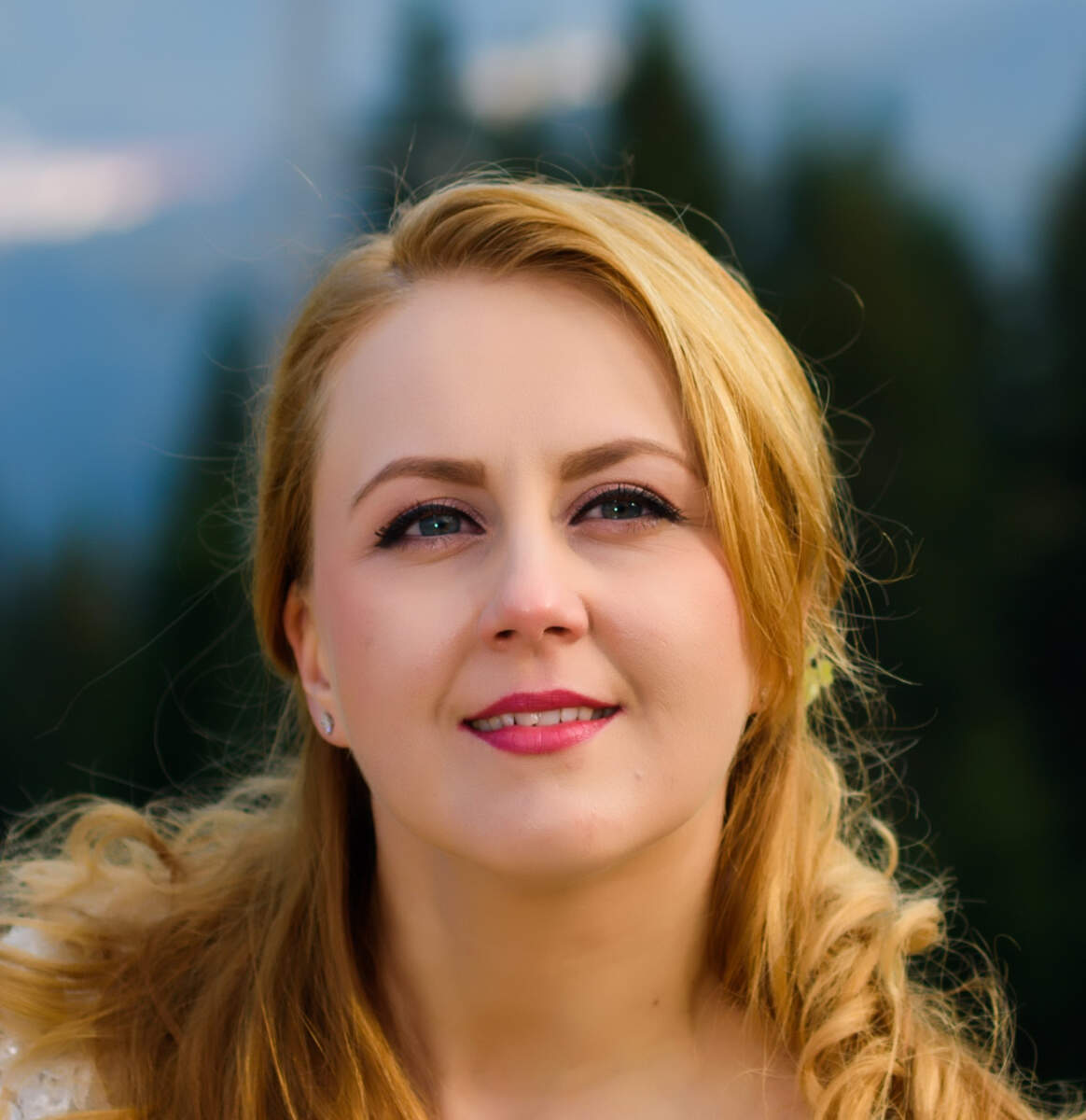
Adriana-Simona Mihaita
Senior Lecturer, Lead of Future Mobility / Lab Data Science Institute, University of Technology in Sydney
Dr Mihaita is a Senior Lecturer at the University of Technology in Sydney, founder and leader of the Future Mobility Lab.
She is an industry-focused academic delivering value for people living in smart cities via her strong engagement with the government and several industry partners. Her research focus is to improve people's movement via artificial intelligence in a smart city context.
She is the winner of the 2022 Women in AI Awards – Infrastructure category, a triple finalist in the 2021 IoT Awards – Smart Cities, 2021 ITS Australia for Transport Data Excellence and for R&D Excellence, the winner of the 2018 ITS Australia National Awards for Research Excellence, nominated Transport for NSW Expert panel member, finalist in Smart City Awards 2019, awarded Monash Business School Scholarship for Women in Leadership 2018, nominated for the 2019 Prime Minister's Science Awards, and interviewed by Channel 7 News and The Guardian.
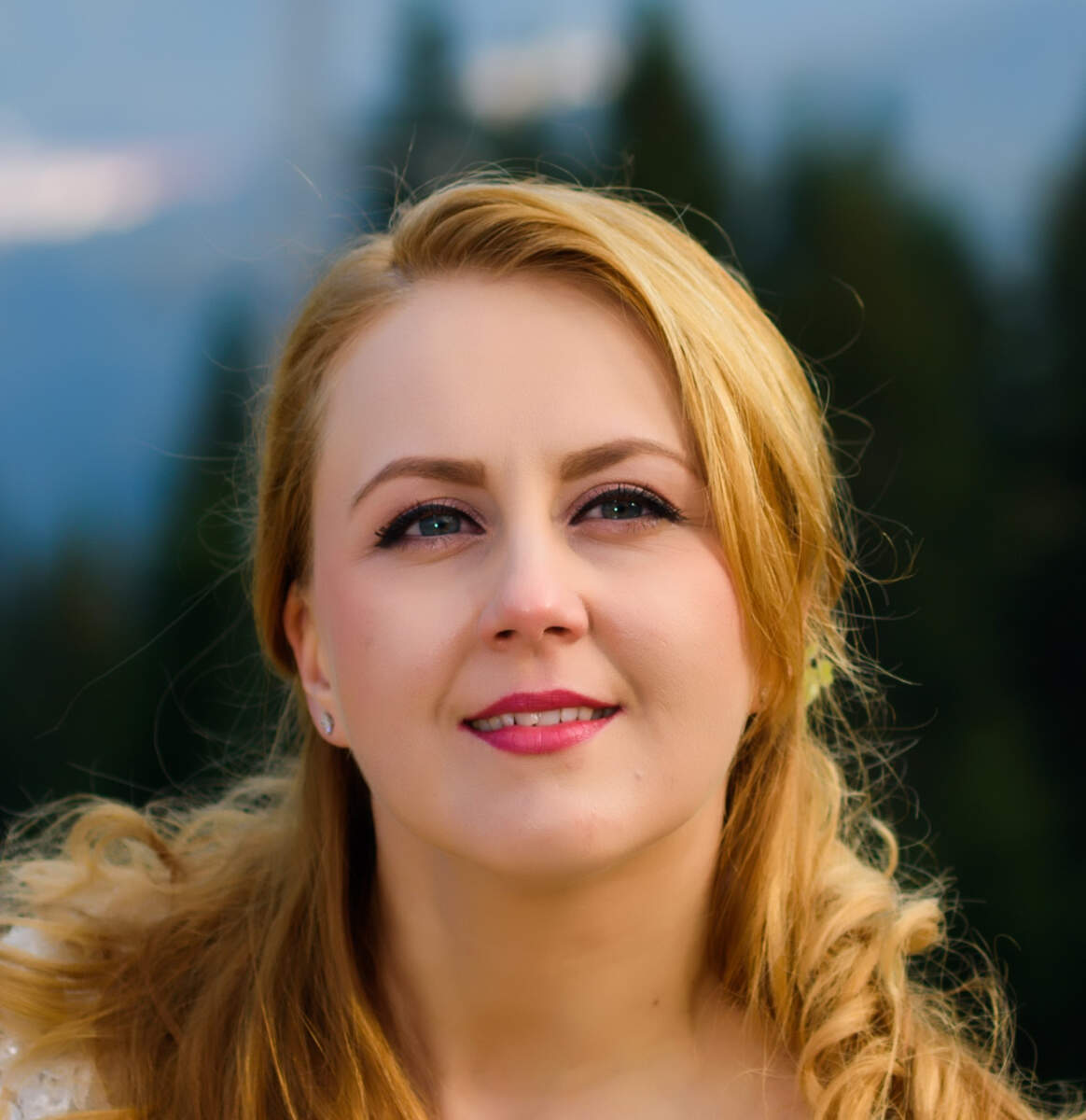
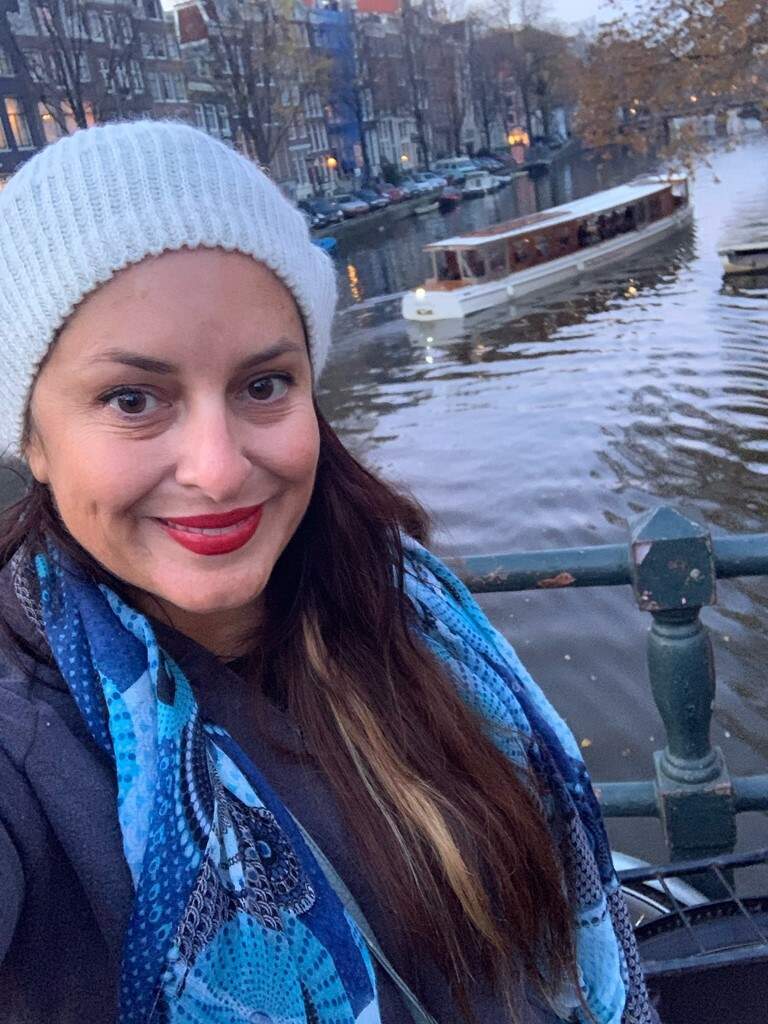
Jennifer Loake
Head of Small & Medium Enterprise Sales – APAC / TomTom
Jennifer leads a team of location technology sales managers across APAC for TomTom. She has 20 years’ experience in location technology having worked in a number of industries including Energy, Resources, and Transportation. Jennifer is focused on contributing towards cleaner and more efficient transportation initiatives in both developed and developing countries. Jennifer holds a Post Graduate Certificate in Management and a Bachelor of Science, both from Massey University in New Zealand.
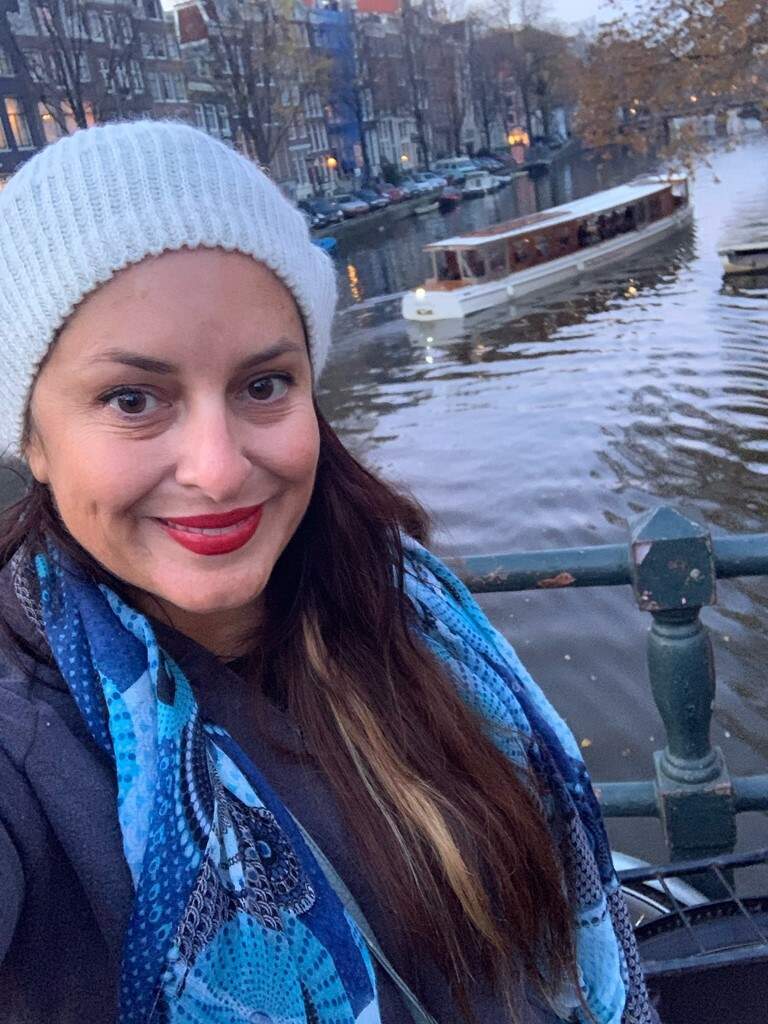
Stacey Ryan: Morning everyone, thanks for joining us. So bright and early. You might have seen Flora just take a photo of all of us that was commemorating. What I'm pretty confident is the first all-female panel for this conference. So hopefully not the last. So, excellent. And this is really the brain’s trust for data. Yesterday we talked a lot about the tech technologies and vehicles and infrastructure that's going to be generating the data this morning. We're going to talk about how we use it, what platforms we can use, what tools are being generated and what other asset owners are delivering data and making sense of it for all of us. So, to that, so it's data driving, clever transport. What does that mean? What are we going to be focusing on? That's what we're going to be hearing from today. So we've got Rita Excel, Head of Transport at AZ at Amazon Web Services, Professor Flora Salim, Professor and Cisco Chair of Digital Transport at University of New South Wales.
Simona Mihaita, Senior Lecturer, Lead of Future Mobility Lab at the University of Technology. Sydney, my alma mater. And Jennifer Loake, Head of small business and medium enterprise Sales APAC at Tom Tom. So we're going to start with Rita. They're going to give us a broad overview of their organizational goals and what they're doing in the field of data application. And what we really want to do for this session, we've got a chunk of time at the end to drive a conversation around what data is available, what we can do with it, and how we can collaborate. Because that's the only way that we can get the benefits of these data that we're generating. So please think of some questions and bring them to these people and make them challenging and interesting, and we'll have a good conversation to kick off the day. Thanks everyone.
Rita Excel: Thanks Stacy, and thanks ITS Australia for inviting me. I'm really thrilled to be here. I want to acknowledge that we're meeting on the land of the Gadigal people, and I acknowledge, leaders, current, past, present, and emerging. So, my name is Rita Excel. I am a transport planner. I've been in, traffic, in traffic and transport and road safety and future mobility for over 30 years and recently I joined AWS, because after six years of working in future mobility and automated vehicles, I realize that data really is going to be, the pinnacle of future mobility. What we do with it, how we collect it, how we store it, and who we share it with. So here's a little bit of an introduction about what we are doing, who AWS is and why we think that now is really the time to leverage the cloud.
I assume everybody knows what the cloud is. I don't want to be preaching about that, but you'd be surprised how many people don't know what the cloud is. We’re more than just storage. We're not just a storage organisation. And, I’ll give you some background to that, but the real reason why people are migrating and organisations have made the decision to move to the cloud, and we top and tell safety and security, I think they're the really the primary reasons, why we come to work, why we exist. We want to be making sure we create a safer environment and also security is, you know, we call it job zero. It's before we start anything, security is really important, but every organisation is modernising. There’s a lot of disruption and transformation happening. So your organisations are looking to modernise. There's a lot of talk about resilience and how we make our, a decision making resilient, how we actually put up with, you know, forces outside of our control, like the ex-Prime Minister of Australia begging everybody in the government.
There's some resilience that we need to build into our own personal, but also professional roles and scalability. If we talk to about apps and people talk about apps, a successful app means that lots of people want to use it. And an unsuccessful app means a lot of people want to use it and nobody can get onto it. So, you know, this whole hyper scaling, having the capacity to do that and having capacity to shut it down, if you know, if you, you are not getting a lot of people using it, that's really the power of the cloud and security, I think I'll talk about security, but security is a shared responsibility. You can't offset your security to somebody else. It's something that you need to take control of. And we'll talk about the philosophy of AWS and security.
So, a little bit about AWS. so, you know, you can't condense experience. My children are 20 and 22. AWS didn't exist when they were born. And I, as I said, I've been in this business for 30 years and it didn't exist when I started and only existed probably when I started my previous job. So in that scale of transport planning and that scale of future, that's a very young organisation, but the enormity and the growth, and the opportunity that it creates, the fact that there's not this locked in deep trenched, entrenched sort of, historical, ways of always doing things, is really one of the benefits of the cloud. But it's also the fear of the cloud as well because it is evolving we have, 31 regions globally, and Australia's one of a handful of countries that have two regions.
And, in January announced our second region in Victoria. So that's a pretty significant thing. And that's on the back of the, the appetite and the investment and the opportunity that the AWS global organisation sees in Australia. and we have, 99 availability zones as well. So this is where, data is stored, data can be stored, and where we have the, a lot of failover opportunities as far as that security scalability for those, that are builders. And I think most of the room here are all builders. we have some clients that are buyers, and some are builders. you know, we have over 200 services that you can start buying your own, whatever your imagination, desires. I'm not going to go into all 200 services, but if you're not familiar with that, you know, it's all available on our website.
So go and have a look at some of the products and services and catch up with the team. We've got a few of the team members here who can give you more details about that. We are really, an amazing thing about AWS and depends on who you work with, but AWS was, designed to help Amazon. So, Amazon, big Amazon, we call it. it's a freight quarter. It's customer centric, it sells things to people. so, it's, we are actually running a business with the services that AWS provides, and that's where AWS spun out of Amazon. We created these things for Amazon about scheduling, being able to get root analysis, being able to make sure we allocate drivers, fleets types of vehicles. So, we we're actually putting into practice the, the sort of, and building the tools using our own products.
So we say that, well, Amazon is our biggest customer of AWS and, not overlooking security. So, AWS, and we'll talk about the security, certifications that we have internationally and also in Australia, and also the shared security model. But why, why cloud? Why for this, you know, for this cloud? Why is cloud important? and we heard a lot, yesterday in the number of sessions around data and data that's coming off of, and this is a particular, case study around the data that's just coming off of the cameras that are located on the road network. Whether it's a tolling managed motorway, whether it's just a monitoring, location at traffic signals. There are so many cameras and this sort of stat is really coming from, the US of the number of cameras that are deployed in the us but it's really critical because there's so much data coming out of these cameras, but we're only really looking at less than 2% of it. So, what can that data tell us? We can talk about real time data, but making real time interventions is really hard. You've got to change all your backend systems, but being a planner at heart, looking at what's happened in the past and being able to make decisions on that to improve it for the future is really important. So, I think there's so much data and we'll hear from the rest of my esteemed colleagues on the panel about what they're doing with data.
I'm not a car freak, but I have spent a lot of time working with OEMs and around autonomous vehicles. But regardless of whether the vehicle's going to be driven by a human or a computer or a robot, vehicles are collecting data and generating data. And so we just really need to understand how we merge, the data that we're getting from our infrastructure from the road and the data that's already been collected by the vehicles and the vehicle manufacturers. So vehicles is an experience now, there’s this term called software defined vehicles in the future where, we already hear about over the air updates and a whole range of other things. So there is so much data being collected by traditional, non-traditional, vehicle manufacturers. And really that's part of the user experience.
So this is a simple point of this is really to say that we've getting so much data from so many different sources, we don't have to generate our own data or collect our own data. It's about how we collaborate on data. And I know, you know, there's a lot of technical people here, so I had to put a diagram of some, you know, architecture up here. This is really about how we are actually creating this connected mobility solution. It's been used by many OEMs around how they ingest the data, what they're getting out of it, and then how they process it back to actually, inform development of their technology. So, the vehicle cycle design cycle use to take years. Now we are seeing that, it can happen more rapidly, and that seems to be what the consumers are looking for.
So, there's many more architecture diagrams around services and products on our website encourage you to go there, particularly for this conference. Tolling and road user charging, the sessions I've been to haven't really focused on road user, road user charging, but that is something that is imminent. It's been imminent for the last 15, 20 years. I think that, you know, that is something we need to have a discussion about and how that works and how that works equitably. And so there's a range of Australia's a really big country and we need to understand how we get information and data, edge computing, how all of these products and services that Amazon provides can be used if, you know, we start having a conversation about what's the architecture behind road user charging and equitable road user charging. So, essentially, when you pay a toll, it doesn't differentiate of whether you are low income, or it, you know, we really don't have that differentiation about how we price things.
So I think it's in the US we're working with, companies like Transurban around how we can actually understand the impacts of tolls and so how to make it more equitable and sustainable. So, we always like to have a case study. And AWS I was really thrilled. I had a US case study here, and then my friends are AMAG, presented yesterday and have a bit of a shout out, to the team here. So this is really bringing the camera solutions or camera data with a service called Panorama from AWS, ingesting that data. And then, providing information back, to a traffic management centre, but also as we heard, storing data for future use and future harassments for planning. So it's great to have an Australian case study. Congratulations to those involved with actually doing these proofs of concepts and having had, you know, elderly parents, having a sister who's living with a disability, just crossing the road with a signal phasing is really a really scary thing for, for them, especially when you're accompanying them.
And, and as a traffic manager, we really want to get the freight moving as fast as we can, and we don't want to have the cues and these pesky pedestrians that take longer to cross the road, you know, mess up the whole system. But it's really important that, with maybe some dynamic, technology like this, we don't have to keep the phase long all the time. We can, change that. So I'm really excited about these sorts of initiatives with our products and I talked about security starting, you know, I want to end on security. It's really something that's top of mind to everybody. We believe in a shared responsibility model around security. If you trust us to put your data into our cloud, you really have to make sure that you still regulate who has access, through your channels into the cloud.
We'll protect the cloud, once your data's in there. But it, you know, Amazon has a principle of providing lease permissions, so you don't provide any permissions unless somebody needs it. And I think that that's really, a model that everybody needs to consider because, encrypting data, all those sorts of things, but bad actors can get into the cloud, and we can't, we don't look at what data you put into our cloud, that's part of the privacy. So if you really, want to learn more about that, it's really important to understand that, Amazon has got a number of certifications globally, with, major governments and in Australia we have an IAP certification for those of you who've been in road safety. It's not the International Risk Assessment Programme but it is, the government's, rating system.
We've been part of that for, for many years now, and we get re-certified all the time. So, that allows governments, and secure data to be hosted in our cloud. So, we're really proud of that, and that's one of the strong credentials we have. We also have, products that help you, to monitor bad actors, attempts on your, on your data. And, we do vulnerability assessments and security assessments and that's something that we can offer our partners and customers. So that's all from me. I look forward to your questions and thanks very much, Stacey.
Stacey Ryan: Thanks Rita. Wise to end on security, I think that's something that's everywhere. It's going to be very much focused on. And I'd like to now introduce, Flora Salim from UNSW.
Flora Salim: Thank you everyone for having me, and it's a privilege to be part of this very first women only panel in this conference. I'm Flora Salim. First, I'd like to acknowledge the Gadigal people and, also leaders past and present.
First, I, I like to say this sessions called data driving clever transport, but I like to take it further. I will say AI driving clever transport. So I have several hats. So I'm a professor of AI and machine learning in the school of Computer Science and Engineering at UNSW. So I'm a computer scientist. I'm also Cisco Chair of Digital Transport, and I'm also, co-leading the transport and mobility focus area in the ARC Centre of Excellence for Automated Edition Making Society. And that's the federal funded centre over seven years that looks at the responsible deployment of ethical automated edition making, including AI and data science systems.
Well, IOT is all over the place, right? In all urban environment, and that everything's being monitored at the moment. So there's a lot of opportunity from, where why we can actually mine from this data. But transport's one of them. But I actually want to say that transport should be the heart of all these things because you can actually use and leverage more. You know, what you can ingest from mobility and transport data to actually inform a lot of decision making in a lot of other areas, including healthcare, including security, surveillance, retail, you name it, right? So I think people in the room regardless whether you industrial PR practitioners or academic or researchers, you are sitting on a goal at a moment but we need to take it further.
And, and this is where my research sits. How do we actually look at and understand behaviour at multiple scale from 3 dimension and individual driving past a toll personalising that to that person to behaviour at a group and even societal scale. And this is my actually interest. How do we actually perform and understand and adapt this behaviour model that is scalable and can be deployed at different areas and ensuring that mobility AI can be not, not just, you know, sits within a as, but how, how do we commoditize mobility AI and put it in the hands of the end users? So this is what I, I like to see in the future and why we need a resilient future. We need our cities to be equitable and sustainable And so, my research is on understanding, learning these complex behaviours, using heterogeneous sensor data in the world.
What I mean by in the world means it doesn't have to be, purposely collected for certain things. You can use different kinds of sensors. Think about your rain gauges to your weather data, to all things to understand people movement behaviour in the city. And we've been working for more than a decade now, and understanding special temporal data from urban sensors from, you have traffic loop detectors to graph data, say transactions between one shop to another shop, how they're related. And all this actually could be informed by this spatial temporal dynamic special temporal data. And this mobile data actually captures dynamics of human behaviour at scale. Now, how do we actually do this in a smarter way? I just want to give you one example. What you see here is a traffic flow forecasting problem, right? So the data that we use are data from Californian Highway and LA and normally what you see is, the fundamental diagram is, you know, you've got traffic density, and as this density decreased, speed will decrease. We all know that. But if you see the, top right that is just an, a visual of every single sensor and every single sensor dynamic, you don't see that, all the time while you see some sensors, you know, even traffic density could be low, you know, the behaviour quite dynamic. So, it could still be slow, so there might be something wrong over there, right? And also because every sensor is unique, every pair is unique, and this happens throughout different times of the day, day of the week, and especially when things go wrong. Now, a lot of AI models can’t cope well with data. They are in the long tail. So, if you know the data and you have your distribution, the long tail AI models often can't cope with that.
A lot of our research in the last five years has been focusing on the long tail and how do we cope with this? So therefore, a lot of our models, also enable long term forecasting because we start ingesting not just your mobility and traffic data, but also a lot of other function functionality data. Again, a lot of these are actually data. We have projects from, in US and also Qatar. So this is a project in collaboration, Qatar Mobility Innovation Centre, where we actually ingested a lot of different data, in terms of even retail people movement. And then this can start informing. If you build a powerful general model, you can then start to customise it downstream. For example, this could be used for air quality prediction. This could be used for parking prediction and this actually enables long-term, not just the next window, prediction, but, several, days ahead.
Now, we need to think about the unprecedented events because of learning climate change our model have to be robust to these things. This is a picture taken from a couple months ago. I think this is from potential, I think it's Kensington Victoria, not here. So the flood, came really, really quickly. Their vehicles driving in, they were trapped in the middle. They could not reverse. Now and, sadly, I've been talking to people from transport here, they said, this no longer becomes just unprecedented. It's going to become more frequent. We have to know, how to deal with this. And the sensor data, as I said, could be quite noisy. And there's a lot of shift in the data distribution behaviour shift. And the problem, again, I like to highlight is a lot of machine learning algorithms you have right now in your toolbox is readily available.
They require high volume of data, and especially training data. What I mean by training data, they need to be properly labelled. We need to think about how do we start relying just on the data that we know is clean, reliable, because we want half the perfect data we want. Therefore, we have been working also on looking at med algorithms that can work with imperfect data, even no label data. So this was a paper and the codes publicly verbal, feel free to use it. How do you do anomaly detection without label data, without you knowing that it's anomaly? We tested this on server data. So, I think it was Amazon or Yahoo server data, I can't remember. But, we could actually predict anomaly detection, on server data, on human behaviour, and also traffic data. This paper that was published last year in triple AI the top conference in AI in the world was actually multi multimodal mobility, now casting during unprecedented societal events.
So the case study was blizzard Jonas. So the snowstorm that disrupted the whole transport network. And you want to predict where will people go, how many people will be in a subway, on a bus, on a taxi, and all those things. And, but how do we know if, because as you can see on the second one, that's the, actually the state of the art model, they fail to predict, things actually going wrong and the error keep going up. Whereas our model, we could pretty close to the ground truth because we have what we call a, a mechanism to be learning in memory. I can talk about the detail further, but please take a look at a paper and further, I want to take, I want to challenge, people in the room here to start also thinking hybrid, not just cloud, but at edge And you can start ingesting modelling on the edge especially.
You know, if you have, if the paradigm you have is self-supervised learning, which means you can learn from its own label rather than trying to collect everything properly, annotate, clean, start on the edge. And when you have ML on the edge, AI on the edge, you can federate the model rather than federating the data, federate the model, exchange the insights you learn from the model you train on the h. Why? Because we have to be prepared for the D-day when things not working, when things are not connected. And finally, I want to say we want to enable this AI model to be used by, you know, people on the street. How do we do that? Nobody, not everyone can code. So, we've been working on this even before ChatGPT was released. ChatGPT like bot for mobility AI.
We've been working with latch language models to ingest mobility data. So, the goal is to have mobility question answering engine. So, we could ask a question to a chat, like, you know, a large language model, how many people will be in central next Sunday? Or, you know, where likely can I get a car park if I want to watch Ed Sheeran, I had a chat with about Ed Sheeran, somebody, last night at the cocktail, when we talk about ticketing, and things like that, right? So, oh, should I take the train? So forecasting is a capability, is, doesn't exist right now in Chat GPT. They try to avoid it, but we've been working with this and I can show you, this is not just a bluff. we have also, two invention disclosures already on this
So, as you know, with the pre-train language model, it's been trained largely on tax quarters. And the power about this is actually then can be used for a lot of different downstream tasks from question answering to information retrieval and so on. What we want to do is we want to be able to customise that and get them to ingest spatial temporal mobility, tr thruster graph whatever data, and it will be able to do Mobility question answering. We have, at least three papers on this topic already. Again, in a top highly selective, AI machine learning conferences, we can turn, oh, the, the, the one of the, recipe about this is mobility prompting. So, we know you, you, whenever you interact with something like your ChatGPT, you prompt, you send a prompt, it will give you an answer.
The same thing with Dall-e, right? You send a prompt to Dali, it will give you an image. Now we do mobility prompting. So, using historical data, you turn this historical data into prompt. and we actually then have a very simple model that has the natural language branch and mobility forecasting, and therefore, the current paradigm on the left, whenever you do any forecasting numbers in, you have encoder, decoder. This is a very simple model. Typically, it's super complex. You saw my paper before with the, you know, long term forecasting. The model could be quite complex because you need to have a specific end quarter for every type of sensor. But what about if you turn this into text, into just unstructured text? you have just end quarter, the quarter, this one here, you can remove that rather. You just use a large language model in the middle, so you can then just prompt and then give you a text answer.
So we, if this is, happening, then definitely everyone on the street could start using it. we have tested this paradigm with large language models like Bert, GPT 2, GPT 3, and we have we just need to create some customised auxiliary tasks to, create some pre-trained masking for the large language models to be able to know that you are now currently predicting about, POI foot traffic, right? So how many people will be in this shop? How many people will be this? you know, and, and there a couple of other things going on at the moment that we are working on to make this automatic, to make this rather than template based by automatic prompting. And I want to give you a couple of examples here.
So we compare ourselves with the just normal, your normal regression algorithm like AMA to transform these methods. They are state-of-the-art. Some of them are award-winning from triple AI last year. and our method consistently performed the best, even beating the models. There are very complex models that could be, that are actually designed specifically just for numerical spatial temporal forecasting. And what is even more interesting, zero short setting. The numbers there are very small, but what I have is a few months of data of people, people movement from location-based services in US. So zero shot setting means I train the data, let's say from NYC. I've never the model, never seen any data from Miami, from Dallas anyway, but we used the model train on NYC to predict, behaviour traffic on the other cities. It worked very well where other models require data to start with. That's why I said AI driving clever transport.
Finally, I want to end on this. We need to be responsible on the way we think about our models. You're seeing that we chat G P D. A lot of people started thinking now, wow, what do we do with our children's education? And some is of these models could be very biased. So are we also working on this on, in the centre of, auto automation making society that I'm co-leading, in this project called transplant Machines? I'm trying to look at how complex deep learning models could be more interpretable. Can it explain the decision outcome? So, the question is, how fair is your model to your user stakeholders? Can we dissect the decision outcome to groups, subgroups of people coming from certain postcode people from another postcode? Is the outcome fair that will be the same as we in the same stakeholder group?
But what about if you have multiple stakeholders, which is common in mobility as a service in road and, and tolling, right? Every single stakeholder will have their own goal and objective of what to optimize. And often they, they're in conflict. This is not a surprise. So as you know, as an operator, you might want to charge the highest, so you couldn't get the maximum profit, but what is it? Is it fair? How do we make sure we can ensure fairness across different stakeholders, while unpacking the biases in this model? So, this is the project we are working on.
So a couple of takeaways I want to throw and questions I have for you, don’t wait until you come up with a perfect data set. Data lake start modelling from now. You can, work with existing AI techniques, or if you can't come and, you know, talk to me, I'll love to collaborate with you. Modelling can start now. Leverage generative AI. So generative AI is becoming more mature, and language models is powerful. And really try to start flipping the paradigm on edge versus cloud. You do need the cloud, right? This is where you have the baseline model, but you also need the edge because then that's where you have things working, even when you don't have any connectivity, when you are in a remote regional area. Think about those, those situations when you have the D-day, the storm, and all those things.
Responsible AI, how robust is your model? How can you ensure the model you deployed will be able to cope with situations that you haven't seen before? And how fair is your model? So that's just a brief snapshot of my group and some of the projects we are working on at the moment. But so my group's called Collective and robust Ubiquitous Intelligence Lab. And I'm also a deputy director of UNSW AI Institute, which is, a whole university institute for on anything related to do with AI covering both cm UNSW, Canberra and UNSW, Sydney also Paddington and Kensington campus. So, if you need, if you have any AI need, definitely, we, there'll be someone, who can, you know, cover your needs because we do also even cover art and design faculty. So, thank you everyone.
Stacey Ryan: Thanks Flora. And ending on that fairness question, I think is very important, sort of that implicit bias baked into those large language models can be a problem. So, I would like to introduce Simona is going to talk to us from UTS.
Adriana-Simona Mihaita: Thank you, Stacey, and good morning everyone. Thank you for getting up early and coming to this panel presentation. So definitely the topic of today's session is about data, how that can be applied to improve our transportation modes. So today I actually have a mix of examples with projects that we have done at UTS regarding transport modelling. I was told to make a short slide about myself, so I did it but anyway, just a short background. I finished automatic control, faculty in Bucharest after that. I did all my PhD studies in France in a control automatic control department in Grenoble and also worked in there, in the university before coming to Australia in 2015, in Data 61 at that time as a research scientist from 2019, I'm in UTS as a senior lecturer where I lead the future mobility lab.
We are working on a mixture of modelling issues that rely a lot on data. So, all the previous speakers have talked about how much data there is. Yes, that's true. but each data can serve a different. So I will just give you some sort of a, a panel of examples, of projects that we have worked upon and, used different types of data. We do actually work on a lot of machine learning, deep learning for traffic disruption. That's my favourite topic in the last seven years, and I'm going to probably chat in the first part a lot about that. Traffic flow prediction arterial motorways, arterial, neighbourhoods, any type of sensors that's produces real time, information with regards to flow, speed, occupancy and so on can be actually fed into an AI and machine learning model in order to be able to produce those short-term and long-term predictions.
Of course, data is not perfect. Flora already mentioned about a long tail distribution, which is quite a challenge. However, if we get reliable dataset, we can have some nice outcomes out of it when data is not available. Our approach is to rely on what we call simulation modelling because these are the ones that really give us the information about what future scenarios, we can take out there. What can we test when we don't have any knowledge about what is going to change in that neighbourhood or in that setting, in that system that we are trying to do. the combination of simulation modelling and, AI or data science you have probably heard about in the last years. The term of digital twin modelling. I will not talk about that today simply because I talked a lot in my previous presentations.
But there is this huge trend of integrating both data driven models that rely purely on, sensing data or any type of real time streaming or historical data, but as well simulation modelling that we need for testing scenarios. Blend this together with the 3D component and you get digital three models that you can do for any type of transportation systems that we currently use, and then work with in our life. Of course, covid disruptions, all sorts, hurricane flooding, long-term, short-term disruptions. This can be modelled as well using that blend of simulation and data driven modelling road safety. we also work with ARAP, TomTom here with regards to an automatic feature extraction from, looking at how we can improve the roadside rating in Australia. and also, you'll hear today a little bit about electric vehicles modelling. Electric vehicles is a different type of beast and requires different type of data and thinking.
So this is yet another, problem that we need to think of how to model air quality can also be embedded smart cities and so on. Anything about control? Alright, there's been a little bit of recognition in what we do, so I will not go over this, but we are happy that, we even have ITS Australia, giving us recognition via awards for our work. So really happy to be continuing working on, data for transfer related problems. The first topic that I really want to, debate a little bit more, as I said, is about traffic incident modelling. Traffic incident modelling. it's something that is very, very difficult for a lot of traffic management centres simply because, there is that unknown situation that is going to happen in order to be able to model that.
After chatting many years with traffic management centres, especially the one in Sydney, we realised that there is not a perfect solution that you can apply in order to be able to evaluate the outcome of an accident. So, this is not an easy problem. You cannot just solve that with just one model, train it, that's it. Accidents can happen anywhere, anytime the stochasticity is really high, randomness can appear anywhere. So it’s a hard problem to solve. That's why we broke this traffic incident modelling into stages the first stage, which is basically re relying on any type of data streams that you have in order to be able to predict, for example, how long that accident will last. And usually that is traffic flow, traffic speed, any type of real time streams that you have and you know, is reliable, you can account on, you can use that data in order to make this predictions on how long accidents will last, five minutes, 10 minutes, 30 minutes, one hour, etc
But once again, when you don't have that reliable data set, when that accident happens in a location that is remotely not covered, you can't really rely on that in order to be able to evaluate the impact of those disruption we go to stage two where we actually do a modelling in space and time of how that incident is going to, propagate into the network. And this relies on a combination of both data driven methods and simulation methods. Finally, the last stage when you really can't rely on your data sets for whatever reason, we usually apply simulation based modelling, in order to be able to do this. So, this is the three stages. These are some examples for each of the stages that I have done with my PhD student, Arthur working on various data sets from both Australia, USA has a rich public open data set in in terms of accidents, in terms of flow. In Australia, there is a lot of reluctance of making incident dataset public, simply because of privacy reasons.
Anyway, we worked on both Australia, US dataset. I will not get into the details, but you have an architecture of multiple machine learning models that have been trained on these three different data sets with intra or extra optimization approaches in order to improve that, duration of how long accident will last. And this also, this is the link for if some of your interests find out more, or please feel free to ask me. Also, we embedded text. So, you know that sometimes operator put text in the incident description saying that oh, two cars collided. Oh, we send one operator to the incident location. We actually employed as well language models that crunch on that text description to improve the machine learning model. So this is another type of data set that we had to blend in into the typical machine learning model in order to improve them.
And it's working. If you have good text descriptions from your operators on the field, you can have some good results. Sometimes it's not that easy. Sometimes what's happening is that you might have a lot of accidents happening at the same time. For this approach, we actually did what we call a traffic accident risk prediction by looking at areas. So it's a different type of, how should I say, problem. We solve this problem by using a kind of similar computer vision approach. And the models we applied in this case is visual transformers, where we simply map out all the accidents and we just divide in grids. The areas where accidents happen, the prediction will be made based on greed-based kind of like approach. So once again, same dataset, but different style of treating the data, different style of getting out the outcomes, and of course proving that is right.
This is just an example of different distributions of sets, as I said, Australia, San Francisco, all of them. and eventually we relied on blending this whole incidents traffic flow, dataset, traffic speed in order to be able to build the best model. And once again, I just want to mention one thing. One model might be good for an Australian neighbourhood, but it might not be good for the San Francisco data set because the characteristics are completely different. So, keep that in mind when you need to build models. Each region, each country is different. The way they store the incidents, the way data is stored is commented, it's completely different. So, we need to work with each data set in order to make the best decisions. I'll try to speed up. This is about, electric vehicle impact modelling as another example where you need to have both data from the energy sector and you need to have data from the transportation sectors and blend those together.
Usually what's happening in, electric vehicles modelling people that are doing the EV uptakes predictions, they simply rely on energy modelling data, that's it, charging data and so on, on the transportation sector, we'd only rely on some simulation modelling. However, nobody really blended these two. I mean, what will be the impact on the energy substation zones if we adopt too many vehicles, too many chargers? We did that. So we basically blended traffic simulation modelling to behavioural modelling and together with energy data in order to build this estimations and impact of, demand in terms of EVs. We put that into a tool and we actually had some cool insights. this is the area where we tested our approach. It's North Sydney. and once again, we had electric vehicle charging stations data coming from those. We had traffic flow counters, data coming from those.
And of course, data coming from AEMO, energy substation zones, all of this, we blended in an architecture. I will not go into all of this. I just want to say that we did the difference between home charging, en route charging and so on. these are some nice videos. Let me see if they play. so basically looking at how can I look at what the traffic volume looks like? These are just small snapshots of the various parts of the project where we are trying to understand where congestion happens and that can actually impact the way people charge. what is the profile of the traffic flow demand during the day, at what time? This is very important for the energy sector. Keep that in mind because of course everybody charging at the same time is going to put a lot of load on the energy network and eventually, other problems can be, explored such as, hey, can we put fast charging in an area?
Well, it depends, and so on whether we have the facility, whether we can actually, afford to change the electricity, demand in that area and so on. So all of this was actually embedded as well in all sorts of different scenarios where we blended the transport and the energy data coming together in order to identify those time of the day profiling. And in order to identify what would be the best time to charge, where are the areas where we need, lots of charging, stations because the demand is simply too high. So all of these dynamic parameters, right, they're simply blended together in order to be able to test and in order to be able to provide, decisions for the City planners. I just have one short example of an Aimsun simulation model. This is once again the stage three.
When you don't have data or when you want to test specific scenarios, you rely on simulation and, it’s really the, typical way to do when you want, for example, to investigate the performance of either your bus network, train network and so on. It's a very powerful tool. you probably, many of you have used it before, which allows you to really implement strategies and blending that together for decision making. I will not insist in this one. My time is almost up. I think the last example is really about motorway flow prediction. It's the simplest type of prediction that we have done. Why? Because all the sensors are in a straight line. usually the time series is very reliable. every three minutes the data comes in. So this was one of those examples where we were so happy because we had a lot of data.
The network was simple. So it was straightforward to train and predict for the next five minutes, 15 minutes, one hour ahead. of course, when disruption happen, accidents happen. It, it was nice to actually see that if you have a very good dataset and you see that highlighted blue square in there, the model actually adjusts, itself to the incoming dataset. So this was happy for us. It was a happy outcome because if you have good and strong patterns into your dataset, reliable dataset, you make robust and reliable models When you don't have that, well, that's a different story. Okay, I will wrap up. other examples of things where we use data really is about, for example, understanding how people behave around train level crossings. I don't know if you have driven in remote areas in Australia or have passed one of those unsynchronized train level crossings.
Be very careful, please. There can be a train coming anytime. A lot of us just assume, what is the likelihood that a train will come Right now it's only 8:00 PM Well guess what? There is a high likelihood, so don't take that for granted. There's a lot of crashes happening around train level crossings. We analyse that data as well in New South Wales and it’s a different type of data that you need to put into it with regards to how people behave. Telematic data anyway. Smart parking, energy integration with transport and driving behaviour. These are just some examples where you can actually model using that data. That's me and thank you so much for your time.
Stacey Ryan: Thank you, Simona. Powered through that. I think it's going to be an interesting challenge. All of these multi-factor, issues that we're, we're seeing I think with the EV charging and deployment is going to be a good one. Now I'd like to introduce Jennifer from Tom Tom
Jennifer Loake: Good morning everyone. Firstly I'd like to introduce myself. My name is Jennifer. I've worked at Tom Tom for five and a half years now. I started out managing a team of engineers. We were working on finding innovative methods to build ETLs to update maps quickly. Now I've moved to the dark side. I'm working in sales, but the part that I love is solving real world problems with technology.
So a little bit about Tom, Tom on where we are right now. So, those who don't know, we were the old portable navigation device company and that's what a lot of people think that we still are, but we're not. We moved to being a software and services company a number of years ago. We've got three key focus areas. We have highly accurate maps, so we are one of four global map suppliers. So there's only four companies or organisations in the world that have a global map. And yeah, we are lucky enough to be one, we've got traffic. So we've worked with UTS with our traffic before. We don't have all vehicles on the road, but we do have around 30% in Australia on the high functional road classes. We also have traffic data, going back historically to 2008. So that's, you know, good data to, test your models out with and train your models with.
Additionally we have APIs. So our APIs help people to answer difficult questions like how long will it take me to get to a certain location, which, which route will I take? We also have SDKs. So navigation SDK is a new thing that we have, which allows you to have turn by turn, navigation instructions, from point A to point B, we've got a new app that's available free in the app store called Amigo. So if you're keen to test out where Tom Tom is at with our navigation, I encourage you to download that. So last year we had a bit of a change. We announced, a new strategy and a new approach. Our focus is on building a new Tom Tom Maps platform, which is application map ready and it allows others to build on top of it. This means that we are looking at, well, we will be decom commoditizing our road centre line. And, this also creates an environment where everyone can build on top and everyone can contribute. So what it does is it builds a, brings a pool of map content from map users around the world and it's going to be the largest geolocation database available in the market. We'll be focused on having continuous updates being fed back into the map, and it will, we hope that it will be the foundation for IOT and connected autonomous vehicle projects to build on top.
So it's going to be built from an array of sources, including osm. So that will allow Tom Tom to have improved coverage. At the moment, we don't support countries like Nepal, Bangladesh, Sri Lanka. from the second half of this year, we will, we'll have, sensor derived observations coming in from vehicles. We'll also have our probe data coming in and we'll have shared POIs. So what will we do? So we will add value by building on top of it ourselves. It’s a lot of data and we'll also be providing data validation from our multiple sources that are coming in and through our technologies. At the heart is a flexible core map. End users can also create private layers where they can hold their own content on top, which can help support their individual needs and use cases. So automating the integration of super sources allows Tom Tom to continuously capture and cons consistently produce a solid base map of the world. But not only that, so towards the latter half of last year, there were a number of press releases, and the Linux Foundation has partnered with Big Tech to develop interoperable and open map data. The Overture Map Foundation is hosted by the Linux Foundation and driven by AWS Meta, Microsoft and Tom Tom.
The ultimate mission of the Overture Maps Foundation is to power new map products through openly available data sets, which can be used and then reused across applications and businesses with each member throwing their own data and resources in the mix. Collaborative map making is central to our strategy at Tom Tom and the Overture Maps Foundation provides us with the framework to accelerate our goals. So in February this year, Esri also announced that they're getting on board and, they joined the Overture Maps Foundation as well. So Esri issued a statement saying, in an increasingly digital and automated world, geospatial data plays a critical role in understanding the physical environment, empowering the next generation of location technologies for geospatial developers and professionals. The ability to access reliable open map data is vital to understanding communities and building innovative services and solutions. So today my presentation is quite short and sweet and I wanted to leave you with a slide and get you to think about what a harmonious open data ecosystem would mean for you. Would there be less system integration costs? Could you have faster build times? Would you feel better for not being locked into one proprietary map vendor?
Would it allow you to have modularity? So I'm leaving you with this thought and welcome any questions. Thank you.
Stacey Ryan: Thank you, Jennifer. And thank you to all of our panellists today. So we've got some time before morning tea and I want to offer the microphones to you to ask some questions, but I'm going to kick that off. I think it's pretty safe to say that this is a daunting, steep learning curve for a lot of folks, especially in the decision making suite. So how do we make life easier in a world where we've already filled with jargon? I spent a lot of my time explaining what an OEM is, let alone some of the more detailed complex things that we're talking about. So in a new world of clouds and lakes and edges and APIs how do we put together an understanding for people that either at beginning of their career or in signing contracts that this is an essential requirement? I'll start with you Rita.
Rita Excell: Thanks Stacey. Look, as I said, I spent 30 years in transport planning, as a civil engineer, and six months ago I moved to AWS. Retraining and understanding some of the IT concepts and emerging, ideas and, and the evolving. Every month there's a new product released. So there's a lot of training tools, self-training, self-paced training that's available. and there's multiple providers that do that. And I would encourage everybody if you are working in this space, to really look at what sort of training you can do. I'm sure my ESTEEM colleagues from the universities, but there's a lot of micro credentials, there's a lot of, professional development that happens. But, it’s really important to look at what training's available out there, up school yourself, up school, your staff, really just to be able to have those conversations and being an informed purchaser. Thanks.
Adriana-Simona Mihaita: I'll say make, make it real. So tell them a story and the current stage and where do you want to bring them next with the new emerging technologies and how it's going to be transforming the, practice and, and then do the deep dive of the technical it ease. and therefore we also focus in a lot of our effort these days on because we use a lot of deep learning. How do we make them explainable? You know, even with this large language model that we've been using for our, for our mobility modelling. They're hardly explainable at all. So we working on that front as well. To ensure the model that we have are robust and trustworthy.
Rita Excell: Outcomes. Focus is really key. Simona.
Adriana-Simona Mihaita: I think my advice would be on two sides. One is on the personal professional preparation side, and one is on the client interfacing problem solving side from a personal preparation, side. What I tend to notice is that, a lot of people tend to jump into directly, you know, using, some models or data science, but they lack the domain knowledge of, the area in which they apply these models. Now, my first advice is first become a very good domain knowledge expert. Like, what area are you working in? Are you working in public transport? Fantastic. Then learn everything about public transport modelling. Are you working in building management? Learn everything about building management first. Have that domain knowledge, which is very hard to have because this will allow you to understand the next problems that you will need to solve.
Computer and data science is usually a tool that will help you to get your destination. It's like a boat, right? But eventually, in order to get that boat, you need to know where you're going. So if you don't know where you're going, doesn't matter what boat you choose, right? So then the second step is once you have that domain knowledge, you understand very good the area, you understand the problems, and you need to know where you will go eventually. It's about choosing, selecting the tools that you need to get you in there. And this can range. And you can learn from experts in pure computer science, experts in algorithms, experts from, cloud service providers that can help you and give you the steps to get there. but eventually know where you need to go. And when you are working on a specific project with a client, I think the most important is to understand what is the problem? Well, what do I, what am I trying to solve? If you are trying, let's say, to solve a pedestrian related problem, why would you kill, your cloud service by loading data about, I don't know, scheduling or something different? Train your models with pedestrian related data. So understand what is the problem that you want to solve? Is it pedestrian? Is it time scheduling? Is it time delay? Is it what I am trying to solve? And then eventually, look at the steps to get there. That's what I would do.
Stacey Ryan: Thanks Simona. And Jennifer, now you're on the dark side selling. How do you make sense of it for?
Jennifer Loake: So, I can tell you a story. When I started at Tom Tom, I came in with about 10 years’ experience in the GIS world. I'd have my own company and I've worked in a lot of different use cases. My first week to two weeks at Tom Tom, I had no idea what anyone was talking about. Too many acronyms, too much difficult language and terminologies. For me it's about demystification, right? Let's make it simple. Let's use less acronyms and let’s, I mean, not everyone is technical that we're going to talk to. We need to be able to engage with high level stakeholders and low-level people, throughout every organisation. So just keep it simple.
Stacey Ryan: Yeah. And I think attaching the solution, solution to a specific area of issue that the people have as well. Like, and, and keeping that simple. So we talk a lot about obviously road safety. It's a critical area for us. So we're going back to the solutions outcomes focused. What are the tools that are available now and emerging that will deliver better road outcomes? Rita?
Well, I’m a heretic in the road safety community because I think the vehicles are really the going to govern road safety, human in the loop - Human makes mistakes. robots can be trained. they can, they're not going to be perfect. but, you know, all of the research shows that, you know, the, the programming a vehicle, robots don't get tired. they generally perform exactly the same way. And that's probably one of the, detriments is that when they have unexpected incursions, they, you know, we really have to train them to be able to predict, not everything's going to be a small child. It might be a leaf. And if you've ever been in a vehicle that stops suddenly, one of the automated vehicles, in the market, that stops suddenly because a leaf flies past you realise the safety critical programming that they have.
So I think, you know, really, understanding how we move and, and, you know, really excited about what the federal governments storing with connected vehicles. We don't have to go to the full spectrum of no driver. We really can start having roads and vehicles talking to each other. How we bring the, the human, into the loop is really important as well. So I think that's really critical from a road safety perspective some of the panels have talked about iRap I was really lucky about 20 years ago to be part of the team with the motoring clubs that bought the AusRap programme to Australia from Europe. that was required a lot of data and that was really a barrier to entry for local government, for others to be able to collect enough data on the road condition to be able to feed into that model. So it's really exciting to see how that's evolved, how we're using data that, you know, third party providers are collecting for other purposes to actually inform road safety. So I think, that the data's really central to decision making, but from road safety, I think we need to see the interface between the vehicles and the infrastructure to really get to that step change.
Stacey Ryan: And Flora and Simone, the models that you're building there're focusing on massive climate disruption and road incidents. Where do you see the future of that with the, like AV’s and more connected vehicles coming on?
Adriana-Simona Mihaita: That's a very hot topic. automation right, and disruption that this will bring. there's a lot to say about automation and how this, what type of data maturity, it will need. from my perspective, I think that, adopting, let's say an autonomous vehicle, transportation system would require a lot of work from the infrastructure level. The infrastructure needs to be equipped with sensors that detect the approaching of those vehicles that respond back, send messages, so that infrastructure to vehicle and vehicle to infrastructure communication needs to go smoothly. otherwise it, it will be very, very hard to adapt to anything that is happening. So first layer would be infrastructure, equipment in order to support the automation level. second level would be to actually facilitate the interaction between autonomous vehicles and all other modes. So all vehicles need to talk to each other vehicle to vehicle, communication in a reliable way. but my feeling is that before we actually get there, we're going to have a mixed transportation mode happening. Autonomous vehicle mixed with personal driven vehicles. I don't think that is going to be a straight directly adoption of avs. AVS will be everywhere. That's, from my point of view, it's going to be a stepping curve and any type of AV system will need to communicate not only with a similar system, but also with human driven vehicles. And that's the challenge. That's going to be the real challenge to equip all vehicles with this capability to communicate.
Stacey Ryan: Yeah, not a bad thing the AVs are a few years off then I suppose, and Flora?
Flora Salim: So, I just want to add to what my colleague here is saying - one of the problems that is still being researched at the moment is, perception, perception of the environment, in even autonomous vehicle environment. And because we as human, as well as we drive, we actually perceive, perceive our environment. And if, if, you know, everything is like, while we are right now human driven, we can still perceive how things are. But when they are actually mixed environment and you, you know, your, our percep level of perception change. And the thing is, there are a couple of things that AI and powerful computation can do better than our human level perception. We need to actually leverage that. We need to enable human’s as they perceive environment, to be able to tap into that knowledge.
But at the same time just like Rita mentioned that we need to actually enable, machines to enable human in the loop. so that kind of mixed environment will be quite interesting when you have perception, especially also a lot of these AV training are trained in a very known control environment. So how would you actually adapt to unknown contexts? you know, suddenly they're, I mean, some of the, vehicles, that are know, are being trained on very flat surface, for example, as soon as it goes to high on, you know, changing elevation, you know, Sydney, it's so hilly like a lot of different places, you won't be able to see the road sign or there's a dog appearing, you know, just, on the side of road it's going to be unthinkable.
Adriana-Simona Mihaita: I just want to wrap up saying data ecosystem eventually for automation, we'll need to get there. We need to have an ecosystem of multiple datas blended together. I don’t know if it's, if anybody's in the room from Telstra or any type of telecommunications company, we need you, that won't be done without you. So it's going to be at another level of data integration.
Flora Salim: Yeah, I agree. And I think one thing to mention as well is the, going back to my presentations, the federated learning. So having a capability of self-contained learning on the edge on each of the vehicle itself, right? But you need, you know, you, you won't get a hundred percent. that's where you, that you need the federated learning where you share the model being learned across between infrastructure, unit to roadside unit, unit to vehicles and, and even, you know, maybe buildings.
Just if I can, just a shout out. I don't know if anybody's here from the Transport for New South Wales customer services team or future mobility team, but there is a survey that they're asking for feedback and they are creating an Australian data set to train models, for automated vehicles. So if you go to the transport from New South Wales website and probably put in Australian data set, they're asking questions like, what sort of road environment should we, what sort of road rules should we be putting into this data model? So shout out to that team that's running that project. So we don't want to have models that are trained overseas, on overseas conditions deployed in Australia, and there isn't an Australian data set for training and assessing automated, technology and perception systems. So those sorts of things that Flora was saying, Australian conditions, bidirectional, you know, dynamic traffic controls, all those sorts of things. Our, our language. We really need to really build the ecosystem and opportunities for Australian companies to design Australian specific tests for these technologies. So, yeah, I encourage you, it closes on Friday. so, get in there and put in your submission
Stacey Ryan: Always comes back to the kangaroos, Jennifer, a lot of this sits on the maps to make it work. How, how are we going to do that?
Jennifer Loake: Yeah, I think it's all around having the ecosystem. I think we've used that word quite a bit today. but I think it's about having people contributing, but people using and making it easy and accessible.
Stacey Ryan: We talked about the training sets. So what are the costs when you talk, looking at building up those training sets and developing a schema, and how do we get to the point where we can have this robust data sets that are easy to use and understandable?
Jennifer Loake: So I think there's always going to be the base level, right? Where we want to be able to make data accessible. And it needs to be free. It needs to be open so that everyone can start having a common, base level, base layer. And, I think anyone who's done system integration between, you know, trying to apply traffic on top of different, base geometries, yeah, it's a, it's difficult. How do you get it down to the lower functional roads? how do you make sure that it's telling you what it, you need it to be telling you a different people model, motorways, roundabouts, all of these different features differently. So standardising that and everyone working off the same their foundation, I think that allows us to then start to innovate above
Stacey Ryan: And Simona and Flora. The models that you are building, say around like road crashes, we don't have a harmonised road crash data set across Australia. So is there like a way to reverse engineer that, like from your side through to the road crash scenario or, in investigator unit
Adriana-Simona Mihaita: It will be so good to have a harmonised crash data set across the entirely of Australia. One thing for us. I'll just touch on the crash data. Transport for NSW might store incidents differently. the operational and intervention on the field are, are completely different. Once again, I mentioned the textual description that operators need to do, and once they get a call and they say, okay, accident on M7, please send two vehicles or crash collide human fatalities, yes or no. So most of the times they don't have time. things happen so far they need to be on, they need to send the intervention team. They don't have time to put all the details inside the database. That's the big issues, which means that many times we have broken records coming in, broken incident logs saying, accident M7 the location, even the location of that accident. It's somewhere in the middle of road, but it's not really exactly precise. So I think improving, first of all, the collection of the crash data from the field would be fantastic to harmonise and uniform all of that. That would be so good.
Stacey Ryan: Yes. I suppose designing tools that would be more efficient to use on scene recovery units. Flora.
Flora Salim: One thing I will say that beyond crashes, what be really, really good is to have a lot more data on near misses. Yes. That's actually the, the critical bit, you know, in what cases crashes will happen and in what cases won. Yes. Because, you know, if you only look at crashes data, then your, your model, whatever you are training on, is very biased on things that eventually did happen. And, it’ll be really good to look at near misses and, yes. Well, my PhD was on looking at, you know, machine learning all those mis near misses or 10, 10 more than 10 years ago now. But even then, you know, a lot of things are have to be simulated.
Adriana-Simona Mihaita: There is a startup company, Compass IOT, if you've heard about them, it's an Australian startup company. They are collecting telematic data from vehicles, mostly the new models that send their position every few seconds. And they are looking at the combination between the steering of the wheel, the speed, the position of the vehicle, and they classify that into near misses or breaking points, all of that. We used them last year, in the last year, they're awesome. Check them out if you haven't. It's Compass IOT.
Rita Excell: Just on that again, not harping on just about vehicles, but there's a lot of data coming from vehicles about hard breaking, those sorts of things. So I think we probably need to start having a conversation about, you know, what data matters from a road safety, from a transport planning, and what's the value of that data and how do we share that data? So AWS, we work with very sensitive data from lots of organisations, and, we’ve got these things called synthetic data sets. So it's actually, getting rid of this whole, it's anonymizing the data. we have these products called clean rooms, which are just announced, late last year. So creates a place where, organisations can share data, and they can have access to it. So we provide the room, for others to collaborate in.
So there's a number of opportunities we just need to maybe start shifting the discussion and I know Australia's been trying to get vehicle generated data consensus on, on how to get that. It's sort of, I'm not quite sure, I've been away from it for a while. But, you know, the ip, the privacy, all those sorts of things. I know in Europe, you know, there are already agreements on what data can be shared around incidents, responses and, and black ice, those sorts of things that the vehicles are detecting. So I think probably we need to have a really mature discussion about what data matters for what purpose, and then also understand there is ip, there is hacking. Vehicle manufacturers don't want, vulnerability into their system. So I think that that's one thing that I would say is really important. And AWS provides, some of these, collaboration opportunities for, sharing data.
Jennifer Loake: Yeah, I totally echo that. I think, we should build upon, what has been done in Europe and then try and, regionalize that. I think, if we start to reinvent from the ground up, it's going to take us a lot longer. We've got to deal with different state jurisdictions in Australia, whereas if we try and have a harmonious approach, I think that's going to allow us to get there faster.
Stacey Ryan: Okay. We've got 10 minutes to go, so if there's any questions, please grab the microphone and ask them. But I'm going to flag one that I think is going to be super easy to answer. How do we get more women in stem?
I think data is a really great anchor. Like when I was at Arup, we used to do lunchtime sessions in Python. We would do crowdsourcing, sort of gamified to your work, India and Nepal and other developing countries. We would, have crowdsourced maps and we would draw octagons around sheds and label parts of the maps so that we could build up a better understanding of, where things were for NGOs. And most of those sessions were filled with young women.
Rita Excell: Yeah, I've got an answer. So, another thing that I've always found is that women do like to talk. and, I find that when you're working in engineering and, and coding and those sorts of things, people put on their headset, turn it onto, aeroplane mode, and there's no conversation. So it's boring. So, for me it's about, creating an energy, creating a hum making it inclusive, and, and really making it fun.
Adriana-Simona Mihaita: Academics
Flora Salim: Such a hard question to ask. How do we get more women in? The answer is by making it look not that hard. It's not that hard if you put your heart, your head to it. Any, you can't do anything. Even if it doesn't matter. You are a female, you're a man, doesn't matter. Eventually, the most important is to be passionate about what you do. And probably we need to, from when they're very young, we need to tell them this. When I started my university degree, we were five women and 100 men in my theory, in the faculty. So, it's 5% women studying automatic control and information technology. I was one of the weird outliers for wanting to study computer science and automatic control, but I loved it. and I was just telling everyone, you can do anything as long as you're passionate about the domain that you're working in, it doesn't matter. So, yeah, that's the message I would encourage from young age.
Stacey Ryan: Yeah. Start that positive reinforcement. Very young.
Flora Salim: First thing I'll say, there's no linear career pathway into stem. You can even be an, a non-technical person and have a career in stem. And even right now just highlighting, the director of National AI Centre, St. Solar, who got, the undergrad training UNSW, but not in AI not in technology, but in arts. So I will say there's not, but the, the most important thing is, reinforce the, positive passion about what you do. and especially if we can start actually showing that STEM is actually fun since, it'll be a bit too late, you know, four kids got in year 11 or 12 because they already decided some of their, courses for, and, you know, what they want to do for hex. But starting early, even the senior primary getting, the kids excited because I, can see, even in UNSW in the moment, some of the struggles I see, with some of the female cohorts are, you know, they feel that they're left behind. But, in comparison to their male, male cohorts who've been coding, because they were coding it while gaming since they were 11, 12 13. So, you know, it's typical to do coding while you're gaming, but the, the female, they, don't game as much and they only start learning coding in, in uni. Sometimes they feel they can't catch up. But it's not, it about, someone who is better in coding, will be better in stem, not necessarily, they'll be a lot of different diverse skill sets that's needed in stem, especially the one I mentioned, responsible ai, responsible data science, responsible innovation. That is a very multidisciplinary discussion. That will need to bring every single skill set from law, from arts, from social science, anthropology, not just the human factors.
Stacey Ryan: And that ties to Jennifer's point as well, that storytelling is going to be vitally important going forward. And women can talk sometime, some of them,
Rita Excell: Just on that, I have to disclose, I have two daughters and neither of them have gone into STEM. So I, you know, as a role model for my own children, but I blame my husband because he is an engineer as well. So it must have been his fault, not mine. In a previous life, I was president of an industry association in local government and we created, an, a scholarship for women to enter and to do post-graduate studies in asset management. I was really excited, really to target, to make sure that, you know, it really targeted women, to be able to apply for this. There was a lot of flak that came. Why is it only targeted at women? You know? So, we're still having this discussion we got some amazing candidates apply.
Some of those candidates probably wouldn't have even considered applying, but because it was specifically targeted, for women, it really started the conversation with their managers with themselves, you know, around accessing that. I do think that, through my children's career at, at high school, they weren't A students in mathematics and chemistry and physics, so they got streamed straight out of that, because it was all about achieving this higher Atar, this magical number around, for the school to be able to brag about, how many people over a percentage. So I think that there's some fundamental issues and maybe we've sold, engineering, and STEM in a wrong way. We've got really intelligent people setting really high bars, but we also have normal people like me, who worked hard and really, you know, worked on the tools and, and, and developed, a career in engineering and I wasn't in the 99 percentile. so I think there's a lot of language, but these fantastic role models that are up here with me, for the next generation, I think that's really important. And making sure we have really good role models for women and also diversity and inclusion, all those things. They're not just words, they're a reality. So, it’s really important for our sector.
Stacey Ryan: Excellent. I think that's a call to action for people at this conference as well. So we want to uplift and have sort of multi-generational people to attend and talk and different folks from different backgrounds. I think that's sort of the next barrier that we need to break through so that we all come from different lived experiences and have different approaches and opportunities and face different challenges so that we understand when we are building these models that, where the biases are, so we get better and fairer outcomes for all of us. So I think that's a good note, but if we've got a question
Audience Member: So I'm Bairgia I'm a PhD student from Monash City at Department of Data Science and AI. So my question is related to Chat GPT like, navigation guidance. So what I want to ask is when chat GPT is used, it is a language based model where we use constraint to analyse the database and provide answers. But when it comes to navigations, most of the data and incidents are real time. So how do you try to process this data? Like for an example, if you want to find a reroute, we have to use a, a dynamic traffic assignment algorithm. So how do you going to integrate this to the suggested system?
Flora Salim: There's a great question. So, you know, there are different power, different AI models and algorithms for different purposes, right? So an example that we show there, it’s basically, a really powerful, language model that can actually be customised for special temporal modelling and forecasting. we show that even when, when you have zero data for training, we can use it for predicting traffic, traffic flow, pedestrian flow at see data that it has never seen before. So, it is really good for those purposes. But for things like, for example, realtime data coming in, we've shown in, briefly, very briefly in the previous, work before I mentioned about the ChatGPT1 one is we need to design a memory model.
That part may not have, have the language model component, but it needs to be able to remember, what if there's any event happening, a similar, so it can then predict even in, during unprecedented events, during incidents or disasters even. and we show that that model that module can actually be adapted to the architecture we have and we haven't tried it, but it may be able to be blocked to the, language model that we have as well. But that's a really good question, but, it’s not that okay, We think language models everything. No, there are different tools for different purposes.
Stacey Ryan: It's good to see chat GPT being used for something good. I didn't think that was going to be possible. can you join me in thanking our panel and, we’ll see you all at morning tea. Thank you.